Oceanography : A remote sensing perspective
Introduction
Oceanography, the study of the physical, chemical, biological, and geological aspects of the ocean, plays a crucial role in understanding Earth's complex marine environment. This field leverages a variety of methodologies and technologies, including remote sensing, to collect, analyze, and interpret oceanic data.Fundamentals of Oceanography
- Physical Oceanography: Physical oceanography deals with the study of ocean currents, waves, and tides. It explores the interactions between the ocean and the atmosphere, which significantly influence climate patterns.
- Chemical Oceanography: Chemical oceanography focuses on the chemical composition of seawater and the biogeochemical cycles. It examines the sources and sinks of various chemical elements and compounds in the ocean.
- Biological Oceanography: Biological oceanography investigates the marine organisms and their interactions with the ocean environment. It covers the study of marine ecosystems, food webs, and the impact of human activities on marine life.
- Geological Oceanography: Geological oceanography examines the structure and composition of the ocean floor. It includes the study of plate tectonics, underwater volcanism, and sediment processes.
Key Remote Sensing Instruments
- Radiometers: Measure the intensity of radiation, providing data on sea surface temperature and ocean color.
- Radar Altimeters: Measure sea surface height, which is crucial for understanding ocean circulation and sea level rise.
- LIDAR (Light Detection and Ranging): Uses laser pulses to measure the depth and topography of the ocean floor.
Oceanography is an interdisciplinary field that utilizes advanced technologies, including remote sensing, to study and understand the ocean. By integrating data from various sources and using sophisticated data formats, oceanographers can monitor, analyze, and predict changes in the marine environment. This knowledge is crucial for managing marine resources, mitigating the impacts of climate change, and protecting ocean health.
Sentinel 3 : Satellite
Sentinel-3 is an European Earth Observation satellite mission developed to support Copernicus ocean, land, atmospheric, emergency, security and cryospheric applications. It is jointly operated by ESA and EUMETSAT to deliver operational ocean and land observation services. There are two satellites lanuched: Sentinel-3A on 16 February 2016 Sentinel-3B on 25 April 2018 (a more detail on the satellite and various components are given at official page).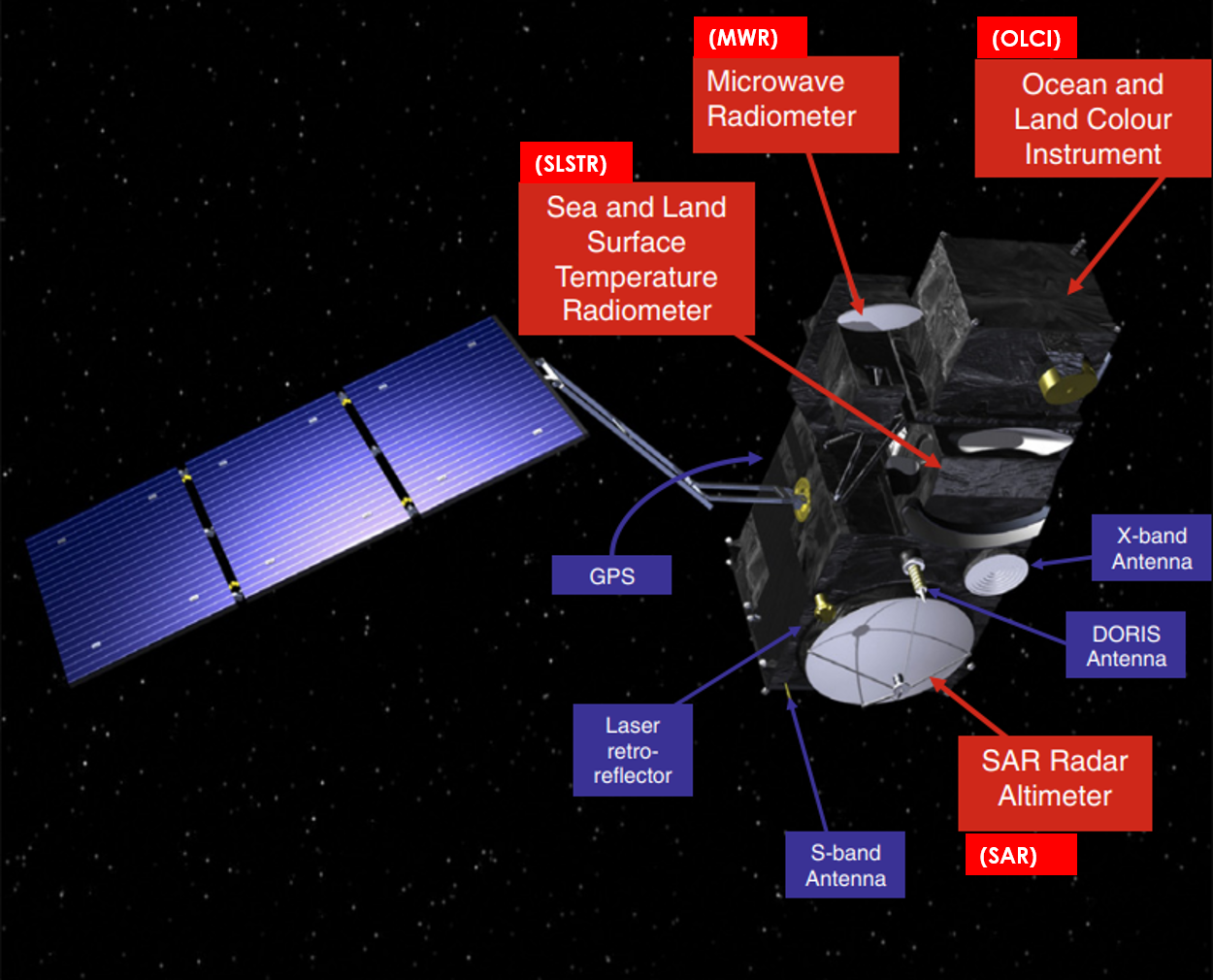
- Main objectives: Systematically measures Earth's oceans, land, ice, and atmosphere.
- Measure sea surface topography.
- Measure sea and land surface temperature.
- Measure ocean and land surface color.
- Additional applications: Sea-level change & sea-surface temperature mapping, water quality management, sea-ice extent and thickness mapping and numerical ocean prediction; land-cover mapping, vegetation health monitoring; glacier monitoring; water resource monitoring; wildfire detection; numerical weather prediction.
- Spectral bands: (For more on theoretical basis on the Spectral Calibration details, see page number 13 in the following link)
Sentinel-3 Instruments:
The Sentinel-3 mission includes several key instruments, each serving distinct functions for Earth observation. Here's a list of the instruments along with a brief explanation of each:- Sea and Land Surface Temperature Radiometer (SLSTR):
- Function: Measures global sea- and land-surface temperatures daily with an accuracy better than 0.3 K.
- Description: This dual-view (near-nadir and inclined) conical imaging radiometer builds on the heritage of the ENVISAT AATSR instrument. It provides accurate temperature measurements essential for climate monitoring and weather forecasting.
- Includes two thermal infrared channels for active fire detection and fire radiative power measurement.
- Supports Copernicus Emergency Response and Climate Services.
- covering 9 spectral bands (550–12 000 nm), dual-view scan with swath widths of 1420 km (nadir) and 750 km (backwards), and a spatial resolution of 500 m for visible and near-infrared, and 1 km for thermal infrared channels.
- S1 (0.555 μm): Cloud screening, vegetation monitoring, and aerosol detection.
- S2 (0.659 μm): Normalized Difference Vegetation Index (NDVI), vegetation monitoring, and aerosol detection.
- S3 (0.865 μm): NDVI, cloud flagging, and pixel co-registration.
- S4 (1.375 μm): Cirrus detection over land.
- S5 (1.61 μm): Cloud clearing, ice, and snow detection.
- S6 (2.25 μm): Cloud clearing.
- S7 (3.74 μm): Surface temperature and active fire monitoring.
- S8 (10.85 μm): Surface temperature.
- S9 (12.0 μm): Surface temperature and emissivity.
- Ocean and Land Colour Instrument (OLCI):
- Function: Captures detailed optical images to monitor ocean and land color, supporting studies of marine biology, water quality, and vegetation.
- Description: This push-broom imaging spectrometer is based on the heritage of the ENVISAT MERIS instrument. It collects data in multiple spectral bands, allowing for comprehensive analysis of oceanic and terrestrial ecosystems.
- Features 21 distinct bands in the spectral region of (400 - 1040 nm).
- Oa01 (400-412 nm): Used for atmospheric correction.
- Oa03 (442-454 nm): Used for chlorophyll absorption peak
- Oa08 (665-677 nm): Used for chlorophyll absorption.
- Oa17 (709-712 nm): Used for detecting fluorescence from chlorophyll.
- Oa21 (1020-1040 nm): Used for atmospheric correction and water vapor content
- Swath width of 1270 km, overlapping with SLSTR swath.
- covering 21 spectral bands (400–1020 nm) with a swath width of 1270 km and a spatial resolution of 300 m.
- Features 21 distinct bands in the spectral region of (400 - 1040 nm).
- Microwave Radiometer (MWR):
- Function: Provides wet atmosphere correction to enhance the accuracy of the altimeter's measurements.
- Description: This instrument measures the brightness temperature at specific microwave frequencies, helping to correct the signal delays caused by water vapor in the atmosphere. It features independent thermal control and cold redundancy for all subsystems to ensure reliability
- Operates in dual frequencies at 23.8 & 36.5 GHz
- 23.8 GHz: Sensitive to water vapor in the atmosphere.
- 36.5 GHz: Used for detecting liquid water content in the atmosphere, surface emissivity, and soil moisture.
- Supports the SAR altimeter.
- Derives atmospheric correction and atmospheric column water vapour measurements.
- Synthetic Aperture Radar (SAR) Radar Altimeter (SRAL):
- Function: Measures sea surface height, wave height, and wind speed over the oceans. Provides accurate topography measurements over sea ice, ice sheets, rivers, and lakes.
- Description: SRAL operates in two frequency bands. This dual-frequency SAR altimeter builds on heritage from the ENVISAT RA-2, CryoSat SIRAL, and Jason-2/Poseidon-3 missions. It provides accurate and reliable altimetry measurements essential for ocean topography and sea state monitoring
- Ku-band (13.575 GHz): Used for high-resolution altimetry over the ocean, coastal zones, sea ice, and inland waters.
- C-band (5.41 GHz): Used for reducing ionospheric delay effects and improving accuracy over different surface types .
- Precise Orbit Determination (POD) Package:
- Components:
- Global Navigation Satellite Systems (GNSS) instrument.
- Doppler Orbitography and Radiopositioning Integrated by Satellite (DORIS) instrument.
- Laser retro-reflector (LRR).
- Function: Ensures high-accuracy radial orbit data, which is crucial for precise altimetry measurements.
- Description: This package combines several technologies to provide precise orbital data, which is necessary for the accurate geolocation of the altimetry data.
- Components:
OLCI module
The Ocean and Land Colour Instrument (OLCI) provides spectral information on the colour of the oceans. It is a radiometer, which measures light reflected by the Earths surfaces. This signal contains a rich wealth of information about the composition of ocean waters, the land surface, and atmosphere. EUMETSAT produces the marine products from the Sentinel-3 OLCI sensors. Ocean colour data provides a window in to the biological activity of the worlds oceans, as well as other oceanographic and coastal processes around sediments and anthropogenic impacts. Sentinel-3 OLCI data can also be used for atmospheric composition applications, including monitoring fires, aerosols, and dust. Current OLCI marine products contain some atmospheric composition related products, and further products are in development. Sentinel-3 OLCI land products are processed by the European Space Agency (ESA).For example, this data can be used to monitor global ocean primary production by phytoplankton, the basis of nearly all life in our seas. Ocean colour data is also vital to understand climate change — ocean colour is one of the Essential Climate Variables listed by the World Meteorological Organization to detect biological activity in the ocean’s surface layer. Phytoplankton take up carbon dioxide (CO\(_2\)) during photosynthesis, making them important carbon sinks.
- Ocean colour data can be used to monitor the annual global uptake of CO\(_2\) by phytoplankton on a global scale. Using this data we can study the wider Earth system, for instance the El Niño/La Niña phenomena and how these impacts the ocean ecosystem. Beyond climate, ocean colour data is also useful to look at more sporadic events.
- OLCI data can be used track sediment transport, monitor coastal water quality and track and forecast harmful algal blooms that are a danger to humans, marine/freshwater life and aquaculture.
OLCI spectrum
The 21 wavelengths used to measure TOA radiance. The measured spectral response functions, which described the true shape of the wavebands, are available for both Sentinel-3A and -3B. The sampling period of the OLCI detectors (CCD’s) runs at 44ms, which correspond to a mean along-track distance of about 300m. Therefore, OLCI produces the Full Resolution (FR) product with a spatial resolution of approximately 300m. In addition, the Reduced Resolution (RR) product is generated with a spatial resolution of approximately 1.2km with 16 (nominally four by four) FR pixels averaged to create a RR pixel; the number of pixels used is varied in the across track direction, as a function of the across track pointing angle from the RR product pixel centre, so that spatial resolution degradation is minimised at the FOV edges.The OLCI bands are available below in the table (taken from OLCI website).
Band number | Central wavelength [nm] | Spectral width [nm] | Function |
---|---|---|---|
Oa1 | 400.000 | 15 | Atmospheric correction, improved water constituent retrieval |
Oa2 | 412.500 | 10 | Coloured Dissolved Organic Matter (CDOM) and detrital pigments |
Oa3 | 442.500 | 10 | Chlorophyll-a (Chl-a) absorption maximum |
Oa4 | 490.000 | 10 | High Chl-a and other photosynthetic pigments |
Oa5 | 510.000 | 10 | Chl-a, TSM, turbidity and red tides |
Oa6 | 560.000 | 10 | Chl-a reference (minimum) |
Oa7 | 620.000 | 10 | TSM |
Oa8 | 665.000 | 10 | Chl-a (2nd absorption maximum), TSM and CDOM |
Oa9 | 673.750 | 7.5 | Improved fluorescence retrieval |
Oa10 | 681.250 | 7.5 | Chl-a fluorescence peak |
Oa11 | 708.750 | 10 | Chl-a fluorescence baseline |
Oa12 | 753.750 | 7.5 | O2 (oxygen) absorption and clouds |
Oa13 | 761.250 | 2.5 | O2 absorption band and AC aerosol estimation |
Oa14 | 764.375 | 3.75 | AC |
Oa15 | 767.500 | 2.5 | O2 A-band absorption band used for cloud top pressure |
Oa16 | 778.750 | 15 | AC aerosol estimation |
Oa17 | 865.000 | 20 | AC aerosol estimation, clouds and pixel co-registration, in common with SLSTR |
Oa18 | 885.000 | 10 | Water vapour absorption reference band |
Oa19 | 900.000 | 10 | Water vapour absorption |
Oa20 | 940.000 | 20 | Water vapour absorption, linked to AC |
Oa21 | 1020.000 | 40 | AC aerosol estimation |
OLCI processing baseline
The OLCI operational processor comprises three major processing levels: Level-0, Level-1 and Level-2 (for more details, please check the link):- Level 0: This step processes raw data contained in instrument source packets. Level 0 products are internal products, and are not disseminated to users.
- Level 1: This step processes the level 0 data to geo-located and calibrated top of atmosphere radiances for each OLCI band.
- Level 2: This step processes the level 1 data to water leaving reflectances and bio-optical variables (eg chlorophyll-a concentration).
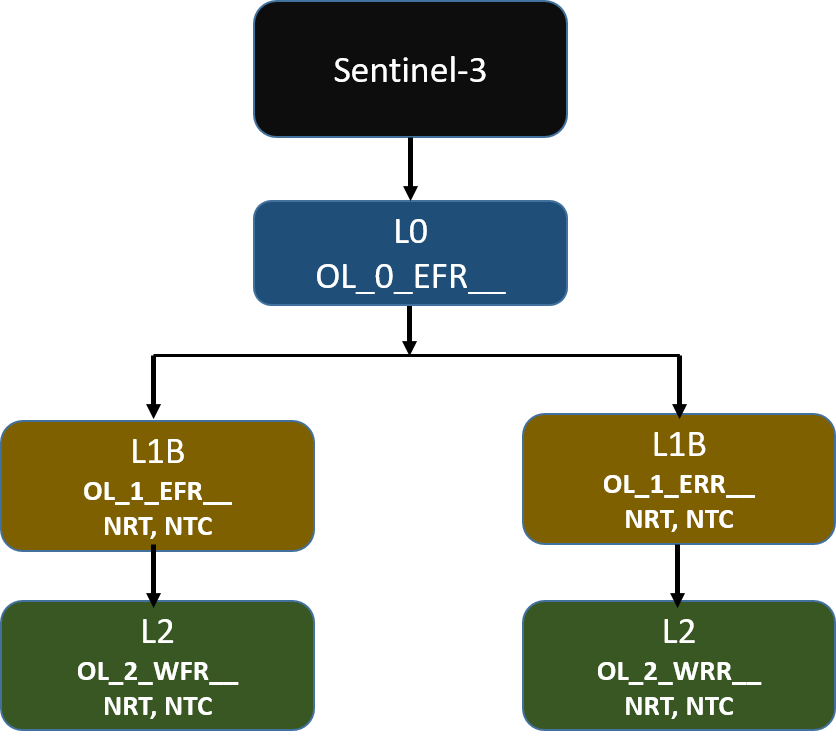
The OLCI level 1B processor operates in three different modes, each creating specific types of products:
- Radiometric Calibration Mode:: Processes calibration data to develop radiometric models (used to measure the intensity of light). The radiometric model is built using continuous in-flight calibration data. It includes: Radiometric Gain Coefficients: These are reference values that help convert raw data into accurate measurements, updated regularly to ensure accuracy. Long-term Evolution Model: This model tracks and adjusts for changes over time.
- Spectral Calibration Mode: Processes calibration data to develop spectral models (used to measure the light's wavelength).
- Earth Observation Mode: Produces detailed and location-specific Earth observation data at full and reduced resolutions.
OLCI Product
Data from all the Sentinel satellites operated under the European Commissions Copernicus Programme are delievered in "SAFE format". The Sentinel-SAFE format is a specific variation of the Standard Archive Format for Europe (SAFE) format specification designed for the Sentinel satellite products. It is based on the XML Formatted Data Units (XFDU) standard under development by the Consultative Committee for Space Data Systems (CCSDS). Sentinel-SAFE is a profile of XFDU, and it restricts the XFDU specifications for specific utilisation in the Earth Observation domain, providing semantics in the same domain to improve interoperability between ground segment facilities.
Each product package includes:
- a manifest file containing a metadata section and a data object section (an xml file).
- measurement data files (NetCDF-4 format)
- annotation data files, if defined (NetCDF-4 format)
The product package can exist as a directory in a filesystem, zipped folder or tarball. The containing unit has a set file name that describes the product.
The Sentinel-SAFE product package file naming is based on a sequence of fields (for more details, please check official documentation):
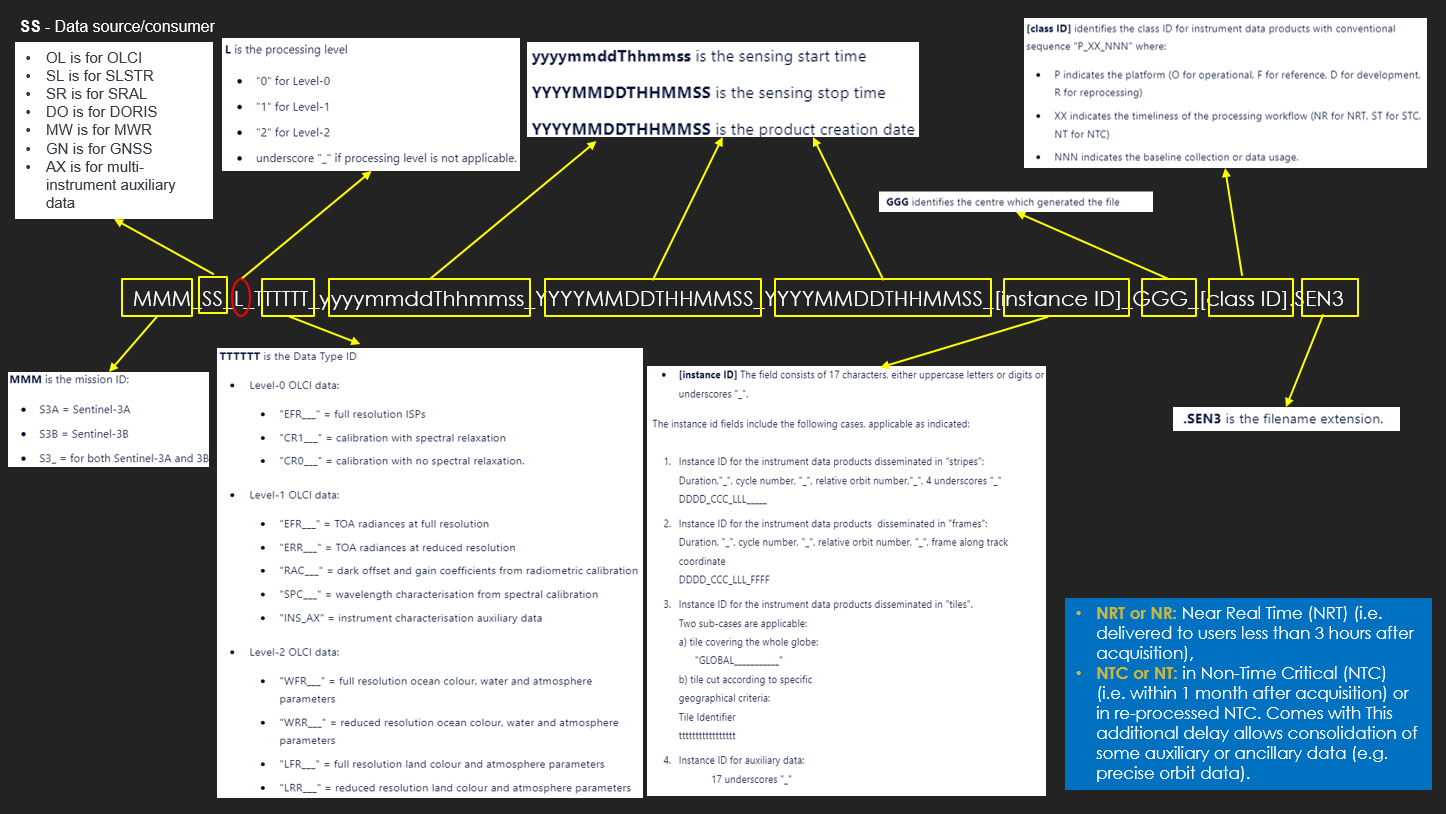
For example, Sentinel-3 near real-time OLCI marine data at Level-2 product file looks like: S3B_SL_2_AOD____20210803T215713_20210803T220308_20210804T002802_0355_055_229______MAR_O_NR_001
, where:
- S3B for Sentinel 3B
- SL for SLSTR data source
- L=2, for level 2 data
- 20210803T215713: Sensing start at 03 Aug 2021 at 21:37:13 hr.
- 20210803T220308: Sensing stops at 03 Aug 2021 at 22:03:08 hr.
- 20210804T002802: Product created at 04 Aug 2021 at 00:28:02 hr.
- 0355_055_229____ (17 character): 'Duration'_'cycle-number'_'relative orbit number'____
- GGG–Product Generating Centre:
- 'MAR' for Marine Processing and Archiving Centre (EUMETSAT).
- 'LN3' for Land Surface Topography Mission Processing and Archiving Centre.
- SVL = Svalbard Satellite Core Ground Station.
- O_NR_001 (format 'P_XX_NNN'): Eight characters to indicate the processing system. So here O=operational, NR for near real-time. Three letters/digits ('001') indicating the baseline collection. A significant change typically triggers a reprocessing and a new baseline collection.
OLCI Sentinel-3 data (analysis)
Accessing OLCI data via the EUMETSAT Data Store
Product Description | Data Store collection ID | Product Navigator |
---|---|---|
Sentinel-3 OLCI level-1B full resolution | EO:EUM:DAT:0409 | link |
Sentinel-3 OLCI level-2 full resolution | EO:EUM:DAT:0407 | link |
Sentinel-3 OLCI level-2 reduced resolution | EO:EUM:DAT:0408 | link |
The project directory is as follows:
EUMETSAT-courses/
│
├── env/
├── Sentinel-3_OLCI/
│ ├── 1_OLCI_introductory/
│ │ ├── products/
│ │ │ ├── S3A_OL_1_EFR____20210717T101015_20210717T101315_20210718T145224_0179_074_122_1980_MAR_O_NT_002.SEN3/
│ │ │ │ ├── geo_coordinates.nc
│ │ │ │ ├── Oa01_radiance.nc
│ │ │ └── S3A_OL_2_WFR____20210717T101015_20210717T101315_20210718T221347_0179_074_122_1980_MAR_O_NT_003.SEN3/
│ │ │ ├── chl_nn.nc
│ │ │ └── geo_coordinates.nc
│ │ ├── 1_1a_OLCI_data_access_Data_Store.ipynb
│ │ ├── 1_1b_OLCI_data_access_HDA.ipynb
│ │ ├── 1_2_OLCI_file_structure.ipynb
│ │ ├── 1_3_OLCI_coverage.ipynb
│ │ ├── 1_4_OLCI_bands_imagery.ipynb
│ │ ├── 1_5_OLCI_radiance_reflectance_spectra.ipynb
│ │ ├── 1_6_OLCI_CHL_comparison.ipynb
│ │ └── 1_7_OLCI_light_environment.ipynb
│ │
│ ├── 2_OLCI_advanced/
│ ├── frameworks/
│ │ └── config.ini
│ └── environment.yml (conda) or requirments.txt (with pip)
│── AUTHORS.txt
├── CHANGELOG
├── .gitignore
├── .gitmodules
├── README.md
└── LICENSE.txt
In the Python ecosystem, managing packages and environments efficiently is crucial for maintaining a clean and reproducible workflow. Two of the most popular tools for these tasks are Conda
and pip
. While they both serve to manage packages, they have different strengths and use cases.
- Conda: Conda is an open-source package and environment management system that originally catered to Python but now supports multiple languages. Conda operates seamlessly on Windows, macOS, and Linux, making it a versatile tool for developers working across different operating systems. Conda installs precompiled binary packages, which reduces the need for compiling code during installation. This feature is particularly useful for scientific computing packages that have complex dependencies. Conda automatically resolves dependencies, ensuring that all installed packages are compatible with each other. This minimizes the risk of dependency conflicts.
- Create a new environment: To construct the environment, in terminal/command prompt navigate to repository folder you want to create the environment, and use command to create the pytho environment using:
conda create -n myenv
- Activate an environment:
conda activate myenv
- Install a package:
conda install package_name
- Export an environment to a file:
conda env export > environment.yml
- Create an environment from a file:
conda env create -f environment.yml
-
- Create a new environment: To construct the environment, in terminal/command prompt navigate to repository folder you want to create the environment, and use command to create the pytho environment using:
- Pip: pip is the package installer for Python, and it connects directly to the Python Package Index (PyPI), where the majority of Python packages are hosted. pip is widely used in the Python community and is supported by almost all Python projects and libraries, ensuring broad compatibility. pip's simplicity and straightforward command structure make it an easy-to-use tool for managing Python packages.
- Set up virtual python environment: Similar to pip, following command can be used to create the python virtual enviroment:
python -m venv env
and to activate it on:- In Mac Os:
source env/bin/activate
- In windows using Command Prompt:
env\Scripts\activate
- In windows using powershell:
.\env\Scripts\Activate.ps1
- In Mac Os:
- PyPI Integration: pip connects to PyPI, giving you access to a vast repository of Python packages. This makes it easy to find and install the libraries you need.
pip install requests
- Install a package:
pip install package_name
- List installed packages:
pip list
- Create a requirements file:
pip freeze > requirements.txt
- Install packages from a requirements file: pip supports requirements files (requirements.txt), which allow you to list all your project dependencies. This makes it easy to share and replicate environments.
pip install -r requirements.txt
- Set up virtual python environment: Similar to pip, following command can be used to create the python virtual enviroment:
Conda vs. pip: Which Should You Use?
While both Conda and pip are valuable tools, they serve slightly different purposes and can often be used together:- Conda is best for creating isolated environments and managing complex dependencies, especially for data science and scientific computing projects.
- pip is ideal for installing and managing Python packages from PyPI and is straightforward for general-purpose Python development.
Using Conda and pip Together: Many developers use Conda for environment management and pip for accessing the extensive range of packages on PyPI. Here’s a workflow combining both tools:
- Create and activate a Conda environment:
conda create -n myenv conda activate myenv
- Install packages using pip within the Conda environment:
pip install requests
In the present case, to create the environment, run: conda env create -f environment.yml
. This will create a Python environment called cmts_learn_olci. The environment won't be activated by default. To activate it, run: conda activate cmts_learn_olci
.
- Step- 1: Importing libraries
import configparser # a library that allows us to parse standard configuration files import IPython # a library that helps us display video and HTML content import os # a library that allows us access to basic operating system commands like making directories import json # a library that helps us make JSON format files import shutil # a library that allows us access to basic operating system commands like copy import zipfile # a library that allows us to unzip zip-files. import eumdac # a tool that helps us download via the eumetsat/data-store
- Step- 2: Sometimes we use configuration files to help us set some notebook parameters. The box below reads a configuration file to help us decide how large to make the videos displayed below
THis script check for configuration file and read if it exists in the current working directory.# Define the path to the configuration file config_file_path = os.path.join(os.path.dirname(os.getcwd()), "frameworks", "config.ini") # Check if the configuration file exists and read it if it does if os.path.exists(config_file_path): config.read(config_file_path)
os.getcwd()
: Returns the current working directory.os.path.dirname
: Returns the directory name of the pathname.os.path.join(path, *paths)
: Joins one or more path components intelligently.os.path.exists(path)
: Returns True if the specified path exists, False otherwise.
- Step- 3: Creating a download directory for the data files:
download_dir = os.path.join(os.getcwd(), "products") os.makedirs(download_dir, exist_ok=True)
- Step- 4: Data access: The Data Store is EUMETSAT's primary pull service for delivering data, including the ocean data available from Sentinel-3 and OLCI. Access to it is possible through a WebUI, and through a series of application programming interfaces (APIs). The Data Store supports browsing, searching and downloading data as well as subscription services. It also provides a link to the online version of the EUMETSAT Data Tailor for customisation. To access Sentinel-3 data from the EUMETSAT Data Store, there are following methods that can be used:
- EUMETSAT Data WebUI
- EUMETSAT Data Access Client (`
eumdac
`)
Other way of accessig the data from the EUMESAT using the REST API are as follows:In order to allow us to download data from the Data Store via API, we need to provide our credentials. We can do this in two ways
- Option-1: either by creating a file called `
.eumdac_credentials
` in our home directory (recommended) - Option-2: or by supplying our credentials directly in this script.
Option-1: creating `
For most computer systems the home directory can be found at the path \user\username, /users/username, or /home/username depending on your operating system. In this file we need to add the following information exactly as follows;.eumdac_credentials
` in our home directory.
You must replace '{ "consumer_key": "your_consumer_key", "consumer_secret": "your_consumer_secret" }
your_consumer_key
' and 'your_consumer_secret
' with the information you extract from https://api.eumetsat.int/api-key/ You will need a EUMETSAT Earth Observation Portal account to access this link, and in order to see the information you must click the "Show hidden fields" button at the bottom of the page.. # Get the home directory of the current user home_directory = os.path.expanduser("~") # Construct the full path to the '.eumdac_credentials' file credentials_path = os.path.join(home_directory, '.eumdac_credentials') # Print the full path print(f"The full path to the '.eumdac_credentials' file is: {credentials_path}")
This code will generate the
Note: your key and secret are permanent, so you only need to do this once, but you should take care to never share them Make sure to save the file without any kind of extension. Once you have done this, you can read in your credentials using the commands in the following cell. These will be used to generate a time-limited token, which will refresh itself when it expires..eumdac_credentials
in the home directory of computer system.Instead of saving the credentials into the home directory of the computer systems, we can also save it to a
.env/
folder which should be kept secret by adding the file name to '.gitignore
' file. To create.env
, usemkdir -p .env
, then move the.eumdac_credentials
file into the.env
from the home or create a new.eumdac_credentials
into the.env
. Follwoing python code can be used to create it into.env
:import os import json import eumdac # Define the path to the '.eumdac_credentials' file in the '.env' directory project_directory = os.path.dirname(os.path.abspath(__file__)) # Get the directory of the current script env_directory = os.path.join(project_directory, '.env') credentials_path = os.path.join(env_directory, '.eumdac_credentials') # Read the credentials from the file with open(credentials_path) as json_file: credentials = json.load(json_file) token = eumdac.AccessToken((credentials['consumer_key'], credentials['consumer_secret'])) print(f"This token '{token}' expires {token.expiration}") # Create data store object datastore = eumdac.DataStore(token)
Option 2: provide credentials directly You can provide your credentials directly as follows;
token = eumdac.AccessToken((consumer_key, consumer_secret))
Note: this method is convenient in the short term, but is not really recommended as you have to put your key and secret in this notebook, and run the risk of accidentally sharing them. This method also requires you to authenticate on a notebook-by-notebook basis.
Since, I have used so many data products, I receive following output:datastore = eumdac.DataStore(token) for collection_id in datastore.collections: try: if "OLCI" in collection_id.title and "EO:EUM:DAT:0" in str(collection_id): print(f"Collection ID({collection_id}): {collection_id.title}") except: pass
So, for;Collection ID(EO:EUM:DAT:0410): OLCI Level 1B Reduced Resolution - Sentinel-3 Collection ID(EO:EUM:DAT:0556): OLCI Level 2 Ocean Colour Full Resolution (version BC003) - Sentinel-3 - Reprocessed Collection ID(EO:EUM:DAT:0557): OLCI Level 2 Ocean Colour Reduced Resolution (version BC003) - Sentinel-3 - Reprocessed Collection ID(EO:EUM:DAT:0407): OLCI Level 2 Ocean Colour Full Resolution - Sentinel-3 Collection ID(EO:EUM:DAT:0408): OLCI Level 2 Ocean Colour Reduced Resolution - Sentinel-3 Collection ID(EO:EUM:DAT:0409): OLCI Level 1B Full Resolution - Sentinel-3 Collection ID(EO:EUM:DAT:0577): OLCI Level 1B Full Resolution (version BC002) - Sentinel-3 - Reprocessed Collection ID(EO:EUM:DAT:0578): OLCI Level 1B Reduced Resolution (version BC002) - Sentinel-3 - Reprocessed
- OLCI Level 1B Full Resolution: we want
collection_id
: EO:EUM:DAT:0409. - OLCI Level 2 Ocean Colour Reduced Resolution: we want
collection_id
: EO:EUM:DAT:0408 - OLCI Level 2 Ocean Colour Full Resolution: we want
collection_id
: EO:EUM:DAT:0407
- Step- 5: The products we need for current OLCI data analytics:
We need a number of OLCI level-1b and level-2 products. These products are shown below in a python 'list' called 'product list'. We will retrieve these products from the Data Store.
product_list = [ 'S3A_OL_1_EFR____20210717T101015_20210717T101315_20210718T145224_0179_074_122_1980_MAR_O_NT_002.SEN3', 'S3A_OL_2_WRR____20210717T095732_20210717T104152_20210718T152419_2660_074_122______MAR_O_NT_003.SEN3', 'S3A_OL_2_WFR____20210717T101015_20210717T101315_20210718T221347_0179_074_122_1980_MAR_O_NT_003.SEN3' ]
- Step- 6: Getting the data (Level 1B): We can access the data using either collection id or product id:
# With the level-1B `collection_id` to the `datastore` object to choose the correct collection. collection_id = 'EO:EUM:DAT:0409' selected_collection = datastore.get_collection(collection_id)
With the product name:
selected_product = datastore.get_product(product_id=product_list[0], collection_id=collection_id)
Once we have collection id and product names, we can download the data from the EUMESAT data store:
with selected_product.open() as fsrc, open(os.path.join(download_dir, fsrc.name), mode='wb') as fdst: print(f'Downloading {fsrc.name}') shutil.copyfileobj(fsrc, fdst) print(f'Download of product {fsrc.name} finished.')
where:
os.path.join(download_dir, fsrc.name)
: Constructs the full path for the destination file by combining download_dir and the name of the source file (fsrc.name).mode='wb'
: Opens the destination file in write-binary mode, which is necessary for copying binary data.fdst
: A file-like object representing the destination file that is being written to.shutil.copyfileobj(fsrc, fdst)
: Copies the content from the source file (fsrc) to the destination file (fdst). This function reads from fsrc and writes to fdst until the end of the source file is reached.
Finally, we have downloaded the data in the
products/
folder. Output of the above code is:Downloading S3A_OL_1_EFR____20210717T101015_20210717T101315_20210718T145224_0179_074_122_1980_MAR_O_NT_002.SEN3.zip Download of product S3A_OL_1_EFR____20210717T101015_20210717T101315_20210718T145224_0179_074_122_1980_MAR_O_NT_002.SEN3.zip finished.
The L1B level product is downloaded as a zip file, so lets unzip it and remove the zip.
with zipfile.ZipFile(fdst.name, 'r') as zip_ref: for file in zip_ref.namelist(): if file.startswith(str(selected_product)): zip_ref.extract(file, download_dir) print(f'Unzipping of product {selected_product} finished.') os.remove(fdst.name)
> Unzipping of product S3A_OL_1_EFR____20210717T101015_20210717T101315_20210718T145224_0179_074_122_1980_MAR_O_NT_002.SEN3 finished.
- Step- 7: Level 2 data products: We can now check the products directory to see that we have the level 1B file. Now, lets pull all the parts together and download both level 2 files in a single loop.
This script downloads products from a datastore using specified collection IDs, extracts the relevant files from the downloaded zip archives, and then deletes the zip files. The steps ensure that only necessary files are extracted and stored in the download_dir.collection_ids = ["EO:EUM:DAT:0408", "EO:EUM:DAT:0407"] for product_id, collection_id in zip(product_list[1:],collection_ids): print(f"Retrieving: {product_id}") selected_collection = datastore.get_collection(collection_id) selected_product = datastore.get_product(product_id=product_id, collection_id=collection_id) with selected_product.open() as fsrc, open(os.path.join(download_dir, fsrc.name), mode='wb') as fdst: print(f'Downloading {fsrc.name}.') shutil.copyfileobj(fsrc, fdst) print(f'Download of product {fsrc.name} finished.') with zipfile.ZipFile(fdst.name, 'r') as zip_ref: for file in zip_ref.namelist(): if file.startswith(str(selected_product)): zip_ref.extract(file, download_dir) print(f'Unzipping of product {fdst.name} finished.') os.remove(fdst.name)
Querying OLCI file structure (Level-1B)
To query the data, we will first need to provide the path to the data.# selecting SAFE directory SAFE_directory = os.path.join(os.getcwd(), 'products', 'S3A_OL_1_EFR____20210717T101015_20210717T101315_20210718T145224_0179_074_122_1980_MAR_O_NT_002.SEN3')
Next, we'll create another variable that takes this path, and finds and adds on the names of the manifest file within the SAFE folder. For Sentinel-3, this manifest is always called xfdumanifest.xml
and contains very useful information about the nature of the contents of the SAFE format product.
# selecting SAFE manifest
SAFE_manifest = glob.glob(os.path.join(SAFE_directory, 'xfd*.xml'))[0]
Then, we'll read the manifest file in to a Python object format called a dictionary. This will make it easier for us to interact with the product in the next step.
References
Some other interesting things to know:
- Visit my website on For Data, Big Data, Data-modeling, Datawarehouse, SQL, cloud-compute.
- Visit my website on Data engineering